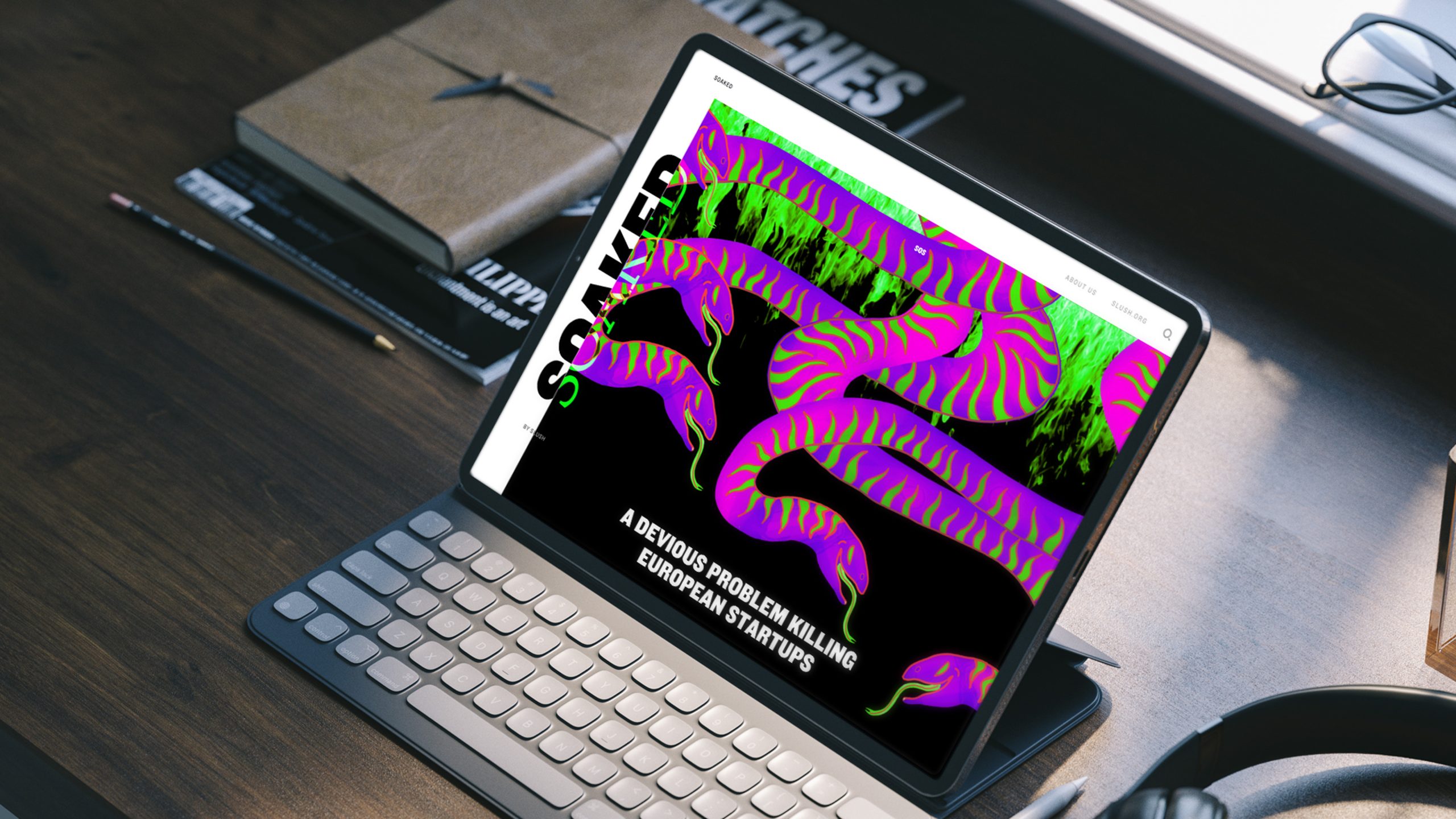
Design
Our designers at Evermade are a blend of creative force and analytic thinking. We sit at the center of design, technology and data. We are born digital and we breathe the Internet. With a roster of global clients, we always crave for the hardest problems to solve.
Principles
① Creativity and outside-of-the-box ideas drive us forward
② Usability, readability, accessibility — we design services for real humans
③ We work heart-to-heart with our in-house developers
What we do
Digital strategy & creative concepts
Our strategy and concept-driven approach powers our work from start to finish. It enables us to understand every aspect of user journeys and needed touchpoints.
Digital brand identities
With a visual eye and a digital mind, we bring brands successfully into the online world. We shape up existing brand identities and craft them from scratch.
User experience & user interface design
We design services, systems and products. We create world-class solutions built to serve our clients’ business goals.
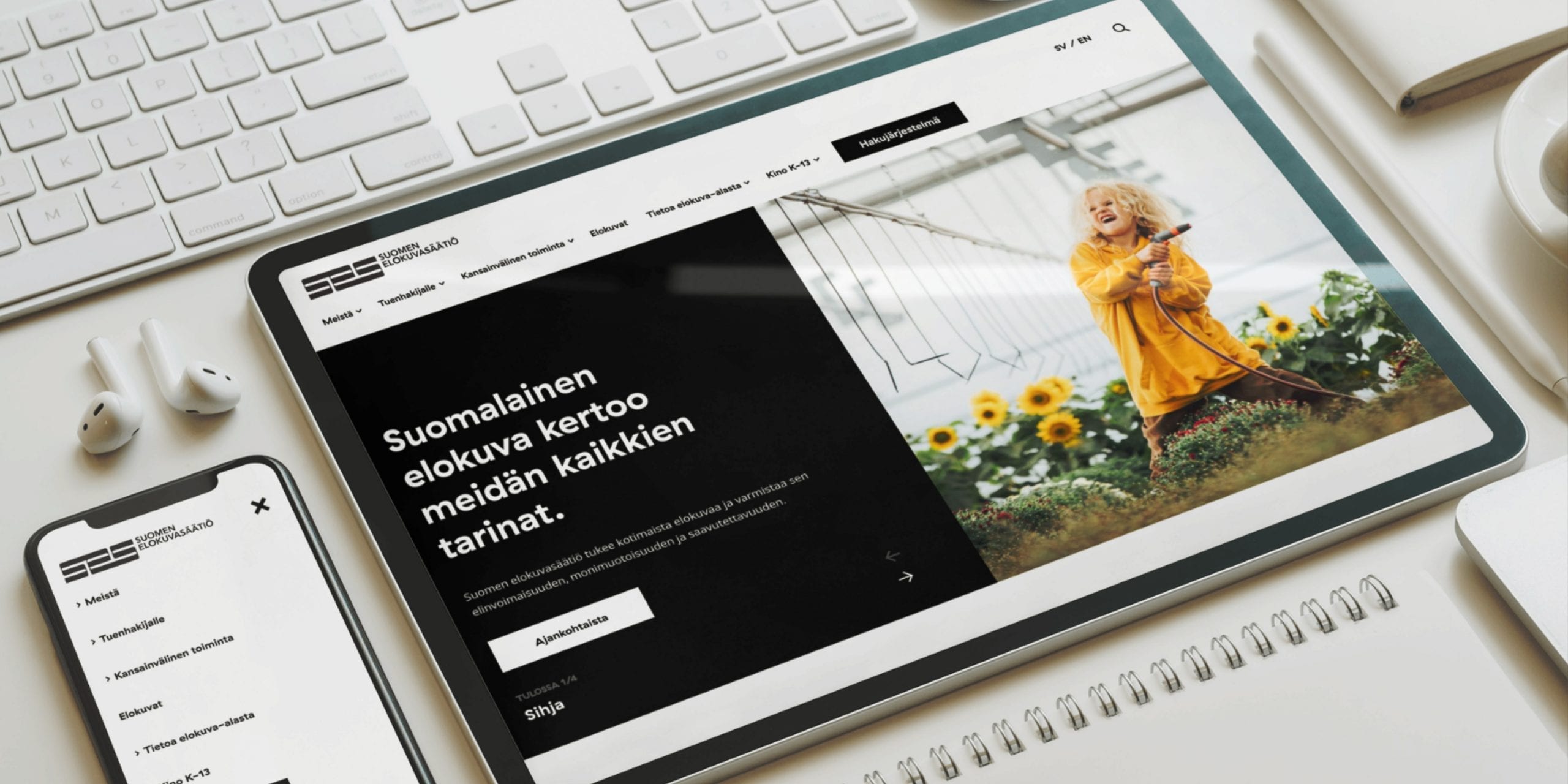
Workflow
Discover
We leave no stone turned. We learn the possibilities and the pitfalls. We form the insight.
Concept
We generate ideas and explore possibilities. We turn insights into tangible concepts and mockups.
Create
We transform prototypes into reality. We master digital experiences, design systems and product design.
Merge
We discuss, iterate and synchronize with developers. We follow the design becoming code and the code becoming a product.
Our top design picks
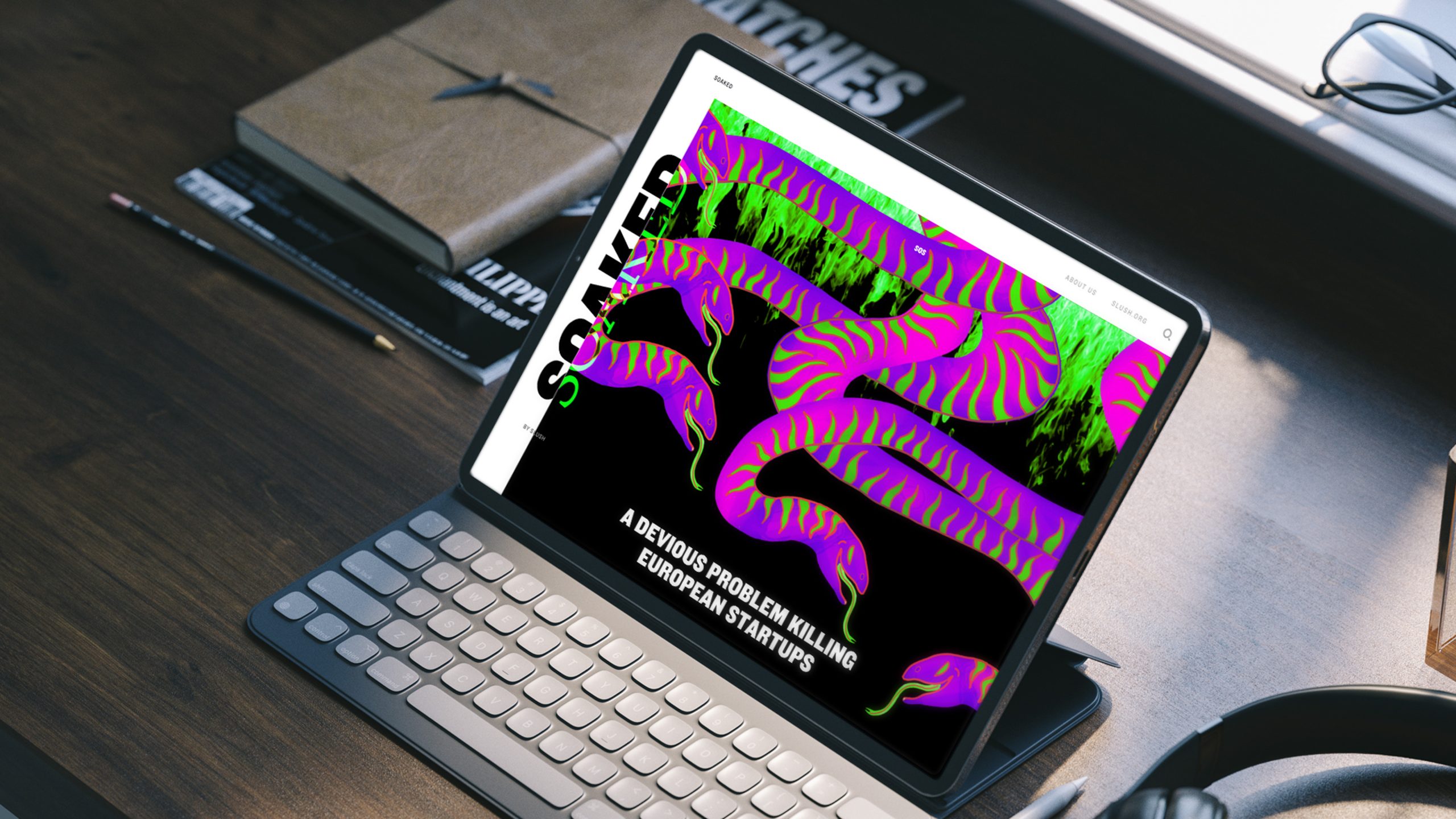